Data exploration stands as the cornerstone of modern analytics, offering invaluable insights that drive informed decision-making and propel businesses toward success. In this comprehensive guide, we delve deep into the realm of data exploration, unveiling its significance, methodologies, and best practices. Join us on this journey as we navigate through the intricacies of data analysis and uncover actionable strategies to harness the power of data.
Understanding the Essence of Data Exploration
At its core, data exploration epitomizes the art of discovery within datasets, allowing analysts to uncover patterns, trends, and relationships that may otherwise remain concealed. It serves as the initial phase of the data analysis pipeline, paving the way for subsequent processes such as data cleansing, modeling, and interpretation. By immersing ourselves in the intricacies of data exploration, we lay the foundation for robust insights that drive strategic decision-making.
The Methodology Unveiled: Techniques and Approaches
1. Exploratory Data Analysis (EDA)
Exploratory Data Analysis (EDA) emerges as a fundamental technique in the realm of data exploration, enabling analysts to gain a preliminary understanding of the dataset’s characteristics. Through the visualization of data distributions, correlations, and outliers, EDA empowers analysts to identify key patterns and anomalies, guiding subsequent analytical endeavors.
2. Descriptive Statistics
Descriptive statistics serve as the bedrock of data exploration, offering concise summaries of dataset properties such as central tendency, dispersion, and shape. By leveraging measures such as mean, median, and standard deviation, analysts can gain nuanced insights into the underlying data structure, facilitating informed decision-making processes.
Best Practices for Effective Data Exploration
1. Establish Clear Objectives
Before embarking on the journey of data exploration, it is imperative to define clear objectives and hypotheses that guide analytical endeavors. By aligning exploration efforts with overarching business goals, analysts can ensure relevance and actionable insights that drive meaningful outcomes.
2. Embrace Iterative Analysis
Data exploration is an iterative process, characterized by continual refinement and iteration. By adopting an agile approach to analysis, analysts can iteratively explore, visualize, and validate insights, thereby fostering a dynamic and adaptive analytical framework.
3. Foster Collaboration and Knowledge Sharing
Collaboration stands as a cornerstone of effective exploration, fostering cross-functional engagement and knowledge sharing across diverse teams. By leveraging the collective expertise and perspectives of stakeholders, analysts can enrich the data exploration process and uncover insights that transcend individual biases.
Techniques for Data Exploration
Statistical Analysis
Statistical analysis is a powerful tool for exploring data, allowing analysts to uncover patterns and relationships through mathematical techniques. From descriptive statistics to inferential analysis, statistical methods provide valuable insights into the distribution, variability, and significance of data.
Data Visualization
Data visualization is an essential aspect of data exploration, enabling analysts to communicate insights effectively through charts, graphs, and dashboards. By visualizing data, analysts can identify trends, patterns, and outliers, making it easier to interpret and analyze complex datasets.
Advanced Techniques
Machine Learning and Predictive Analytics
Machine learning and predictive analytics are revolutionizing the field of data exploration, allowing analysts to uncover hidden patterns and make accurate predictions based on historical data. By leveraging algorithms and models, analysts can identify trends, forecast future outcomes, and optimize business processes for maximum efficiency and effectiveness.
Big Data Exploration
With the advent of big data, businesses are faced with the challenge of exploring and analyzing massive datasets spanning terabytes or even petabytes of information. Big data exploration techniques, such as parallel processing, distributed computing, and scalable algorithms, enable analysts to extract valuable insights from large and complex datasets efficiently.
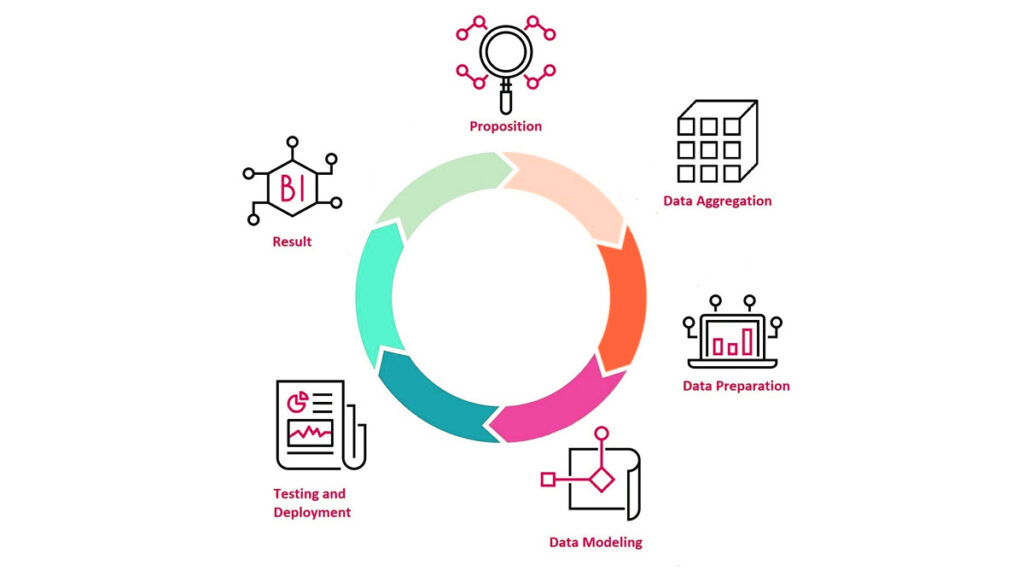
Challenges and Considerations
Data Quality and Consistency
One of the primary challenges in exploration is ensuring the quality and consistency of the data. Poor data quality, such as missing values, outliers, and inaccuracies, can undermine the integrity of the analysis and lead to erroneous conclusions. Therefore, it’s essential to prioritize data quality assurance measures to ensure reliable insights.
Ethical and Privacy Considerations
In an era of increasing data privacy concerns, businesses must navigate ethical and legal considerations when exploring data. From ensuring compliance with regulations such as GDPR to safeguarding sensitive
FAQs
What is the primary goal of data exploration?
The primary goal of data exploration is to uncover patterns, trends, and relationships within datasets to inform decision-making and drive business growth.
How does data visualization enhance the data exploration process?
Data visualization enhances the exploration process by providing visual representations of data, making it easier to identify patterns, trends, and outliers.
What are some common challenges in exploration?
Common challenges in data exploration include data quality issues, ethical and privacy considerations, and the complexity of analyzing large and diverse datasets.
How can businesses ensure the quality and consistency of their data for exploration?
Businesses can ensure the quality and consistency of their data for exploration by implementing data quality assurance measures, such as data cleaning, validation, and documentation.
What role does machine learning play in data exploration?
Machine learning plays a significant role in exploration by enabling analysts to uncover hidden patterns and make predictions based on historical data.
Why is it essential for businesses to define clear objectives before embarking on data exploration?
Defining clear objectives before embarking on exploration helps businesses focus their efforts, prioritize their analyses, and maximize the value of their findings.
Final Wording
Data exploration is a dynamic and iterative process that empowers businesses to unlock valuable insights from their data. By leveraging techniques such as exploratory data analysis, statistical analysis, and machine learning, organizations can uncover hidden patterns, identify opportunities, and make informed decisions that drive success. As we continue to navigate the ever-evolving landscape of data analytics, mastering the art of exploration will be essential for staying competitive and unlocking the full potential of data-driven decision-making.